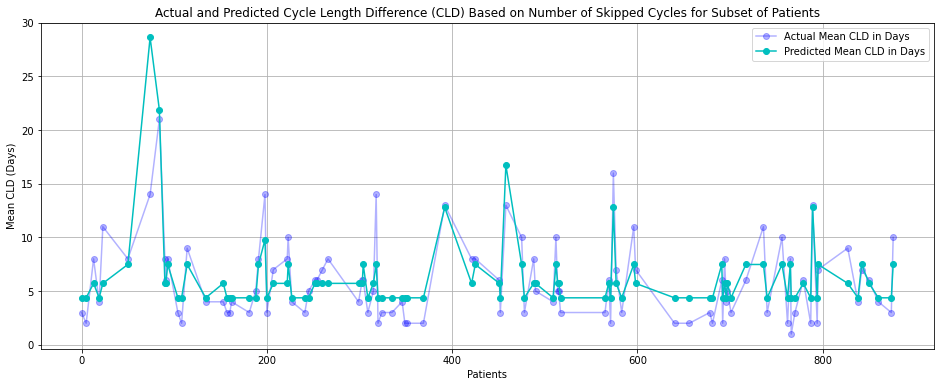
Plot of predicted cycle length differences (CLD) in turquoise and real CLD in blue.
CLD is a measure of cycle length variability.
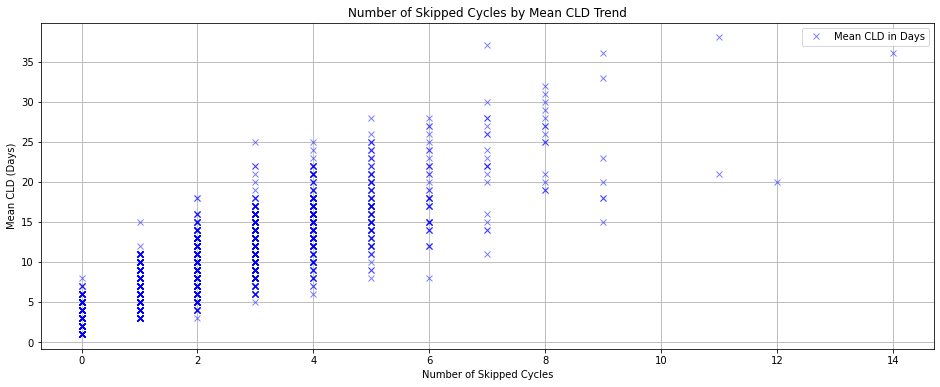
Plot of mean CLD by number of skipped periods.
For this project, I applied a Poisson Regression Model to predict menstrual cycle length variability based on number of skipped periods. Fertility tracker devices and standalone menstrual cycle apps have become popular digital health solutions for people preventing or planning a pregnancy. However, their clinical fertility prediction accuracy is often below industry standards and susceptible to research bias. One approach to address this issue and improve a model's robustness involves identifying additional parameters with direct correlation to menstrual cycle length. This project was created to do just that and investigates whether skipping periods, a common practice among oral birth control users, could be a predictor of menstrual cycle length variability.
Plot of predicted cycle length differences (CLD) in turquoise and real CLD in blue.
CLD is a measure of cycle length variability.
Plot of mean CLD by number of skipped periods.